by Kevin R. Guidry, PhD
Methodological notes: This is not a comprehensive or rigorous literature review. I began by first identifying the most highly cited literature relevant to higher education. I did this in October 2024 by searching for "ai literacy" in Web of Science and ordering the results by number of citations. After reading and summarizing the most highly cited and relevant articles, I then searched for articles that cite and meaningfully build on those highly cited articles, especially those in the higher education context and with a focus on assessment or evaluation instruments.
Defining AI literacy
The first major, recent paper is Long and Magerko's CHI 2020 paper What is AI literacy? Competencies and design considerations This link will take you to an external website in a new tab.. This computer science conference paper reviewed interdisciplinary literature, including "grey literature" that is not peer-reviewed such as publicly-available course syllabi, to define AI literacy competencies for learners. This review resulted in the creation of a conceptual framework with five themes each with associated competencies. The complete list of 17 competencies can be found in appendix A. Other literature (e.g., Laupichler et al., 2022, discussed below) describes this paper as a seminal paper in this rather young field of study with its framework being very influential on subsequent work.
The second major, recent paper is Ng et al.'s 2021 Computers and Education: Artificial Intelligence "exploratory review" of AI literacy This link will take you to an external website in a new tab.. This paper explicitly focused on using available literature to conceptualize AI literacy in a manner that it could be defined, taught, and evaluated. These authors reviewed peer-reviewed literature in the field of education published from 2016 to 2021. From the 30 articles that were finally selected for inclusion in their review, these authors identified the four common aspects of AI literacy in Table 1. Ng et al. also noted that 8 of the 30 articles in their review explicitly borrowed ideas from "computational thinking," a related set of ideas that are broader in focus. In 2014, the University of Delaware Faculty Senate added computational thinking to the university's general education objectives.[1]
Aspect | Definition |
Know & understand AI | Know the basic functions of AI and how to use AI applications |
Use & apply AI | Applying AI knowledge, concepts and applications in different scenarios |
Evaluate & create AI | Higher-order thinking skills (e.g., evaluate, appraise, predict, design) with AI applications |
AI ethics | Human-centered considerations (e.g., fairness, accountability, transparency, ethics, safety) |
Table 1: AI literacy aspects in Ng et al. (2021)
Ng et al.'s exploration of how AI literacy was taught in the studies they analyzed focused primarily on K-12 education so it is of limited utility for educators in the postsecondary context. However, their analysis of how AI literacy was evaluated in those studies seems relevant. In brief, they found a wide variety of methods, including both quantitative and qualitative methods. Instruments that are potentially relevant are discussed later in this brief literature summary.
The third major, recent paper[2] is "Artificial intelligence literacy in higher and adult education: A scoping literature review" by Laupichler et al. This link will take you to an external website in a new tab. These authors applied seven exclusion criteria to narrow their review to 30 articles that specifically focused on AI literacy in higher or adult education, including "grey literature" that nevertheless has reasonable scientific merit (e.g., theses, dissertations) published after 2000. Among their observations are (a) literature specifically about this topic in the postsecondary and adult education context appears to be lagging behind the broader collection of literature in this area by a few years, (b) literature in this area originates more from the US and Asia than other parts of the world, (c) there is significant variance in the quality of published research in this area. Most importantly, these authors found that AI literacy was not yet defined consistently with alternative ideas such as "AI readiness" and "AI capabilities" offered by a minority of researchers.
The fourth major, recent paper is Southworth et al.'s 2023 "Developing a model for AI Across the curriculum: Transforming the higher education landscape via innovation in AI literacy" This link will take you to an external website in a new tab. that describes the comprehensive work underway in AI literacy at the University of Florida (UF). Their university-wide work[3] focuses on teaching AI literacy throughout their undergraduate programs, work that is the focus of the Quality Enhancement Plan required by their institutional accreditor, the Southern Association of Colleges and Schools Commission on Colleges. The UF AI literacy model is an iteration of the Ng et al. (2021) model and is described in Table 2. This model is used by faculty and staff, particularly those in the new AI2 Center who coordinate much of this work, to identify "course gaps or needs to ensure AI learning opportunities are available for all undergraduate students." Student learning outcomes for courses that address each category were also developed and these are discussed in the next section.
Category | Description |
Enabling AI | Support AI through related knowledge and skill development (e.g., programming, statistics) and/or contain a lower total AI content of one of the four Core AI Literacy topics |
Know & understand AI | Know the basic functions of AI and to use AI applications |
Use & apply AI | Applying AI knowledge, concepts and applications in different scenarios |
Evaluate & create AI | Higher-order thinking skills (e.g., evaluate, appraise, predict, design) with AI applications |
AI ethics | Human-centered considerations (e.g., fairness, accountability, transparency, ethics, safety) |
Table 2: UF AI literacy model
The fifth and final major paper[4] is an EDUCAUSE working group paper published in October 2024, AI Literacy in Teaching and Learning: A Durable Framework for Higher Education This link will take you to an external website in a new tab.. This paper proposes three related frameworks for Artificial Intelligence Literacy in Teaching and Learning (ALTL), one for students, one for faculty, and one for staff. Each framework includes definitions, competencies, and outcomes in four areas: Technical Understanding, Evaluative Skills, Practical Application, and Ethical Considerations. For example, the elements included for the student framework are shown in Table 3.
Area | Competency | Outcome |
Technical Understanding
| Fundamentals of AI | Students will understand core AI principles, including machine learning, natural language processing, and neural networks. |
Hands-On Experience | Students will gain practical experience with AI technologies through interactive sessions and workshops. | |
Evaluative Skills | Critical Evaluation of AI Tools | Students will critically assess the effectiveness and reliability of various AI tools. |
Assessment of AI Impact | Students will evaluate the impact of AI on their learning and research outcomes. | |
Fact-Checking and AI Hallucination | Students will carefully evaluate AI output for inaccuracies and hallucinations. | |
Ethical Evaluation | Students will understand and evaluate the ethical implications of using AI in academia. | |
Practical Application | Integration into Learning | Students will effectively integrate AI tools into their daily learning activities, including studying, personalized learning, and review of work. |
Research Enhancement | Students will leverage AI tools to enhance their research capabilities. | |
Project-Based Learning | Students will apply AI tools to project-based learning to enhance critical thinking and problem-solving skills in addressing real-world problems. | |
Ethical Considerations | Responsible Use of AI | Students will use AI responsibly, understanding data privacy, AI bias, and ethical implications. |
Development of Personal AI Policies | Students will develop personal ethical AI usage policies. | |
Vigilance in AI Application | Students will remain vigilant in their use of AI tools, addressing ethical concerns proactively. |
Table 3: EDUCAUSE AI Literacy in Teaching and Learning student elements
Assessing or Evaluating AI literacy
In their 2020 paper, Long and Magerko do not address how AI literacy can be assessed or evaluated. They explicitly acknowledge that "research on AI education is still in its nascent stages" (p. 10) and recommend further work into "what the best practices are for teaching AI to a non-technical audience" (p. 10).
In contrast, Ng et al. (2021) explicitly address assessment and evaluation of AI literacy. One of their four research questions directly focuses on this topic: "How do researchers evaluate students' AI literacy skills?" Among the 30 studies they examined, they identified both quantitative and qualitative methods used to evaluate AI literacy. Quantitative methods included pre- and post-knowledge tests and self-reported measures of AI literacy. Qualitative methods included photographs, observations, and interviews. Ng et al. further identified three major types of assessments in the literature: knowledge tests, surveys, and project portfolio analyses and artifact-based interviews. Although they evaluate strengths and weaknesses of each type of assessment and do not make any clear recommendations, they are optimistic that a knowledge test can be developed to reliably and validly assess AI literacy for summative purposes in the k-12 context and for postsecondary students outside of computer science.
Laupichler et al. (2022) do not directly address how AI literacy can be assessed or evaluated. They briefly mention the topic in their discussion of one of their research questions that focuses on courses that attempt to increase students' AI literacy ("What teaching formats and pedagogical structures are used in courses that have the goal to increase AI literacy in higher and adult education?"). However, three of the four authors collaborated on a subsequent paper (Laupichler, Aster, & Raupach, 2023 This link will take you to an external website in a new tab.) that explicitly focused on an assessment scale. Using a "Delphi study" to solicit input from 53 subject matter experts in three iterative rounds of questioning, these authors developed a 38-item questionnaire.
In their 2023 paper, Southworth et al. not only define AI literacy in the context of their work at the University of Florida but they also define student learning outcomes. Their framework includes five AI literacy categories but only four of them are intended to be applied to students. Six student learning outcomes were created and mapped to those four AI literacy categories. These are listed in Table 4. These outcomes are intended to be integrated into courses and assessed using rubrics that are not explicitly identified or described in this paper.
Student learning outcome | AI literacy category |
SLO1. Identify, describe, and explain the components, requirements, and/or characteristics of AI. (Content knowledge and communication) | Enabling AI, Know & Understand AI |
SLO2. Recognize, identify, describe, define and/or explain applications of AI in multiple domains. (Critical thinking and communication) | Enabling AI, Know & Understand AI |
SLO3. Select and/or utilize AI tools and techniques appropriate to a specific context and application. (Critical thinking and content knowledge) | Use & Apply AI |
SLO4. Assess the context-specific value or quality of AI tools and applications. (Critical thinking) | Evaluate and Create AI |
SLO5. Conceptualize and/or develop tools, hardware, data, and/or algorithms utilized in AI solutions. (Critical thinking) | Evaluate and Create AI |
SLO6. Develop, apply, and/or evaluate contextually appropriate ethical frameworks to use across all aspects of AI. (Critical thinking and content knowledge) | AI Ethics |
Table 4: University of Florida AI literacy student learning outcomes
The EDUCAUSE working group paper AI Literacy in Teaching and Learning: A Durable Framework for Higher Education This link will take you to an external website in a new tab. includes competencies, outcomes, and examples assessments for each of its three frameworks. The authors do not present a unified assessment or measurement strategy or instrument; for example, assessment of the student competencies appears to be intended to be embedded in courses and other educational experiences. The elements included for the student, faculty, and student frameworks are included in appendices B, C, and D, respectively.
Beyond the five papers included in the first part of this summary, several other papers describe AI literacy assessment instruments. These are explicitly cited in several of the first five papers with some describing them in detail or building on them in some way.
In 2022, Wang, Rau, and Yuan published a 12-item instrument measuring AI literacy in "Measuring user competence in using artificial intelligence: validity and reliability of artificial intelligence literacy scale This link will take you to an external website in a new tab.." The questions were developed to measure AI literacy in four constructs: awareness, usage, evaluation, and ethics. The authors performed multiple, iterative rounds of work to test their questions and report acceptable reliability and validity results in their tests. Questions asked respondents to indicate their level of agreement with "statements that addressed the behavior, ability, or level of comfort when performing specific AI-involving tasks" (p. 1328) e.g., "I can choose the most appropriate AI application or product from a variety fora particular task" and "I can distinguish between smart devices and non-smart devices." The full list of questions is included in appendix E.
The following year, Laupichler et al. published two papers (Laupichler, Aster, Haverkamp, & Raupach, 2023 This link will take you to an external website in a new tab.; Laupichler, Aster, & Raupach, 2023 This link will take you to an external website in a new tab.) describing the development of their instrument, the SNAIL (Scale for the assessment of non-experts’ AI literacy). This scale is a 39-item questionnaire where respondents rate their own competency using a seven-point Likert scale. Exploratory factor analysis of a pilot administration to 415 participants yielded three factors: technical understanding, critical appraisal, and practical application. This loading and the labels selected by the authors led them to label them the TUCAPA-model of AI literacy. The questions were randomly ordered for each participant in the pilot administration. A complete list of the questions and the factor on which they loaded is included in appendix F.
Additional AI literacy scales are identified and described in Lintner's 2024 systematic review This link will take you to an external website in a new tab. that identified 16 difference scales. Of the 16 scales, three were performance-based and the remaining 13 were self-reported. At the time that Lintner conducted and wrote their review, most of the scales had some initial indicators of quality (e.g., structural validity, internal consistency) but most lacked advanced indicators of quality (e.g., content validity, reliability) and none had been tested for cross-cultural validity or measurement error.
Appendix A: Long and Magerko's AI Competencies[5]
Theme 1. What is AI?
- Recognizing AI: Distinguish between technological artifacts that use and do not use AI.
- Understanding Intelligence: Critically analyze and discuss features that make an entity “intelligent”, including discussing differences between human, animal, and machine intelligence.
- Interdisciplinarity: Recognize that there are many ways to think about and develop “intelligent” machines. Identify a variety of technologies that use AI, including technology spanning cognitive systems, robotics, and ML.
- General vs. Narrow: Distinguish between general and narrow AI.
Theme 2. What can AI do?
- AI’s Strengths & Weaknesses: Identify problem types that AI excels at and problems that are more challenging for AI. Use this information to determine when it is appropriate to use AI and when to leverage human skills.
- Imagine Future AI: Imagine possible future applications of AI and consider the effects of such applications on the world.
Theme 3. How does AI work?
- Representations: Understand what a knowledge representation is and describe some examples of knowledge representations.
- Decision-Making: Recognize and describe examples of how computers reason and make decisions.
Theme 4. How should AI be used?
- ML Steps: Understand the steps involved in machine learning and the practices and challenges that each step entails.
- Human Role in AI: Recognize that humans play an important role in programming, choosing models, and fine-tuning AI systems.
- Data Literacy: Understand basic data literacy concepts such as those outlined in Javier Calzada Prado and Miguel Ángel Marzal. (2013). Incorporating data literacy into information literacy programs: Core competencies and contents. Libri, 63(2). 123–134.
- Learning from Data: Recognize that computers often learn from data (including one’s own data).
- Critically Interpreting Data: Understand that data cannot be taken at face-value and requires interpretation. Describe how the training examples provided in an initial dataset can affect the results of an algorithm.
Theme 5. How do people perceive AI?
- Action & Reaction: Understand that some AI systems have the ability to physically act on the world. This action can be directed by higher-level reasoning (e.g. walking along a planned path) or it can be reactive (e.g. jumping backwards to avoid a sensed obstacle).
- Sensors: Understand what sensors are, recognize that computers perceive the world using sensors, and identify sensors on a variety of devices. Recognize that different sensors support different types of representation and reasoning about the world.
- Ethics: Identify and describe different perspectives on the key ethical issues surrounding AI (i.e. privacy, employment, misinformation, the singularity, ethical decision making, diversity, bias, transparency, accountability).
Programmability: Understand that agents are programmable.
Appendix B: EDUCAUSE AI Literacy in Teaching and Learning student elements
Area | Competency | Outcome | Example assessment |
Technical Understanding
| Fundamentals of AI | Faculty will understand core AI principles, including machine learning, natural language processing, and neural networks. | Faculty will complete courses or training sessions on AI fundamentals, with assessments such as quizzes or projects demonstrating their understanding. |
Application of AI Tools | Faculty will become proficient in using AI tools for their academic and administrative work. | Faculty will develop lesson plans or administrative workflows that incorporate AI tools, demonstrating effective use through peer reviews or presentations. | |
Hands-On Experience | Faculty will gain practical experience with AI technologies through interactive sessions and workshops. | Faculty will complete hands-on projects using AI tools to solve academic or administrative problems, with assessments based on their application and problem-solving skills.
| |
Evaluative Skills | Critical Evaluation of AI Tools | Faculty will assess the effectiveness and reliability of various AI tools critically. | Faculty will design evaluation rubrics for AI tools, apply them in reviewing multiple AI applications, and submit comparative analysis reports. |
Assessment of AI Impact | Faculty will evaluate the impact of AI on their teaching and research outcomes. | Faculty will conduct case studies on AI integration in their work, presenting findings on its benefits and limitations. | |
Ethical Evaluation | Faculty will understand and evaluate the ethical implications of using AI in academia. | Faculty will engage in debates and write position papers on ethical issues related to AI, such as privacy, data security, and bias. | |
Practical Application | Integration into Teaching | Faculty will effectively integrate AI tools into their daily teaching activities. | Faculty will create personalized learning plans incorporating AI tools for their students, with periodic reviews and adjustments based on student progress. |
Research Enhancement | Faculty will leverage AI tools to enhance their research capabilities. | Faculty will complete research projects using AI for data analysis, literature reviews, and the presentation of methodologies and findings with multimodal AI tools. | |
Course Design and Development | Faculty will apply AI tools to project-based learning to enhance students' critical-thinking and problem-solving skills. | Faculty will work on course design projects addressing real-world problems using AI, followed by presentations and peer reviews. | |
Ethical Considerations | Responsible Use of AI | Faculty will use AI responsibly, understanding data privacy, AI bias, and ethical implications. | Faculty will create guidelines for responsible AI use in their courses and conduct peer reviews to ensure adherence. |
Development of Course AI Policies | Faculty will develop ethical AI usage policies for their courses. | Faculty will draft and submit AI usage policies for their courses, with feedback sessions to refine and improve them. | |
Vigilance in AI Application | Faculty will remain vigilant in their use of AI tools, addressing ethical concerns proactively. | Faculty will participate in ongoing discussions and training on their ethical use of AI and ethical dilemmas encountered. |
Appendix C: EDUCAUSE AI Literacy in Teaching and Learning faculty elements
Area | Competency | Outcome | Example assessment |
Technical Understanding
| Fundamentals of AI | Students will understand core AI principles, including machine learning, natural language processing, and neural networks. | Students will complete quizzes and projects demonstrating their understanding of AI fundamentals, such as creating simple machine-learning models, data analysis projects, and personalized AI tools. |
Hands-On Experience | Students will gain practical experience with AI technologies through interactive sessions and workshops. | Students will complete hands-on projects using AI tools to solve academic problems, with assessments based on their application and problem-solving skills. | |
Evaluative Skills | Critical Evaluation of AI Tools | Students will critically assess the effectiveness and reliability of various AI tools. | Students will design evaluation rubrics for AI tools and apply them in reviewing multiple AI applications, submitting a comparative analysis report. |
Assessment of AI Impact | Students will evaluate the impact of AI on their learning and research outcomes. | Students will conduct case studies on AI integration in their studies, presenting findings on its benefits and limitations. | |
Fact-Checking and AI Hallucination | Students will carefully evaluate AI output for inaccuracies and hallucinations. | Students will research and employ specific prompting techniques to reduce hallucinations, then carefully review AI output. Students will submit screenshots of prompting for verification of their work and reflect on the process. | |
Ethical Evaluation | Students will understand and evaluate the ethical implications of using AI in academia. | Students will engage in debates and write position papers on ethical issues related to AI, such as privacy, data security, and bias. | |
Practical Application | Integration into Learning | Students will effectively integrate AI tools into their daily learning activities, including studying, personalized learning, and review of work. | Students will create personalized learning plans incorporating AI tools, with periodic reviews and adjustments based on their progress. |
Research Enhancement | Students will leverage AI tools to enhance their research capabilities. | Students will complete a research project using AI to identify specific types of sources for data analysis so as to complete annotated bibliographies and literature reviews and to present their methodologies and findings with multimodal AI tools. | |
Project-Based Learning | Students will apply AI tools to project-based learning to enhance critical thinking and problem-solving skills in addressing real-world problems. | Students will work on group projects addressing real-world problems using AI, followed by presentations and peer reviews utilizing image, audio, video, and infographic AI tools.
| |
Ethical Considerations | Responsible Use of AI | Students will use AI responsibly, understanding data privacy, AI bias, and ethical implications. | Students will create guidelines for responsible AI use in their studies and conduct peer reviews to ensure adherence. |
Development of Personal AI Policies | Students will develop personal ethical AI usage policies. | Students will draft and submit their AI usage policies, with feedback sessions to refine and improve them. | |
Vigilance in AI Application | Students will remain vigilant in their use of AI tools, addressing ethical concerns proactively. | Students will participate in ongoing discussions and submit reports on their vigilance practices and any ethical dilemmas encountered. |
Appendix D: EDUCAUSE AI Literacy in Teaching and Learning staff elements
Area | Competency | Outcome | Example assessment |
Technical Understanding
| Fundamentals of AI | Students will understand core AI principles, including machine learning, natural language processing, and neural networks. | Students will complete quizzes and projects demonstrating their understanding of AI fundamentals, such as creating simple machine-learning models, data analysis projects, and personalized AI tools. |
Hands-On Experience | Students will gain practical experience with AI technologies through interactive sessions and workshops. | Students will complete hands-on projects using AI tools to solve academic problems, with assessments based on their application and problem-solving skills. | |
Evaluative Skills | Critical Evaluation of AI Tools | Students will critically assess the effectiveness and reliability of various AI tools. | Students will design evaluation rubrics for AI tools and apply them in reviewing multiple AI applications, submitting a comparative analysis report. |
Assessment of AI Impact | Students will evaluate the impact of AI on their learning and research outcomes. | Students will conduct case studies on AI integration in their studies, presenting findings on its benefits and limitations. | |
Fact-Checking and AI Hallucination | Students will carefully evaluate AI output for inaccuracies and hallucinations. | Students will research and employ specific prompting techniques to reduce hallucinations, then carefully review AI output. Students will submit screenshots of prompting for verification of their work and reflect on the process. | |
Ethical Evaluation | Students will understand and evaluate the ethical implications of using AI in academia. | Students will engage in debates and write position papers on ethical issues related to AI, such as privacy, data security, and bias. | |
Practical Application | Integration into Learning | Students will effectively integrate AI tools into their daily learning activities, including studying, personalized learning, and review of work. | Students will create personalized learning plans incorporating AI tools, with periodic reviews and adjustments based on their progress. |
Research Enhancement | Students will leverage AI tools to enhance their research capabilities. | Students will complete a research project using AI to identify specific types of sources for data analysis so as to complete annotated bibliographies and literature reviews and to present their methodologies and findings with multimodal AI tools. | |
Project-Based Learning | Students will apply AI tools to project-based learning to enhance critical thinking and problem-solving skills in addressing real-world problems. | Students will work on group projects addressing real-world problems using AI, followed by presentations and peer reviews utilizing image, audio, video, and infographic AI tools.
| |
Ethical Considerations | Responsible Use of AI | Students will use AI responsibly, understanding data privacy, AI bias, and ethical implications. | Students will create guidelines for responsible AI use in their studies and conduct peer reviews to ensure adherence. |
Development of Personal AI Policies | Students will develop personal ethical AI usage policies. | Students will draft and submit their AI usage policies, with feedback sessions to refine and improve them. | |
Vigilance in AI Application | Students will remain vigilant in their use of AI tools, addressing ethical concerns proactively. | Students will participate in ongoing discussions and submit reports on their vigilance practices and any ethical dilemmas encountered. |
Appendix E: Wang, Rau, and Yuan's AI literacy scale
Construct | Question |
Awareness | I can distinguish between smart devices and non-smart devices. |
Awareness | I do not know how AI technology can help me. (reverse-coded) |
Awareness | I can identify the AI technology employed in the applications and products I use. |
Usage | I can skilfully use AI applications or products to help me with my daily work. |
Usage | It is usually hard for me to learn to use a new AI application or product. (reverse-coded) |
Usage | I can use AI applications or products to improve my work efficiency |
Evaluation | I can evaluate the capabilities and limitations of an AI application or product after using it for a while. |
Evaluation | I can choose a proper solution from various solutions provided by a smartagent. |
Evaluation | I can choose the most appropriate AI application or product from a variety for a particular task. |
Ethics | I always comply with ethical principles when using AI applications or products. |
Ethics | I am never alert to privacy and information security issues when using AI applications or products. (reverse-coded) |
Ethics | I am always alert to the abuse of AI technology. |
Appendix F: Scale for the assessment of non-experts' AI literacy (SNAIL)
Factor | Item |
Practical application | I can tell if the technologies I use are supported by artificial intelligence. |
Practical application | I can name examples of technical applications that are supported by artificial intelligence. |
Critical appraisal[6] | I can explain the differences between human and artificial intelligence. |
Technical understanding | I can explain the difference between general (or strong) and narrow (or weak) artificial intelligence. |
Technical understanding | I can evaluate whether media representations of AI (e.g., in movies or video games) go beyond the current capabilities of AI technologies. |
Critical appraisal | I can name weaknesses of artificial intelligence. |
Practical application[7] | I can name strengths of artificial intelligence. |
Critical appraisal | I can describe risks that may arise when using artificial intelligence systems. |
Critical appraisal[8] | I can describe advantages that can come from using artificial intelligence systems. |
Practical application[9] | I can describe the potential impact of artificial intelligence on the future. |
Technical understanding[10] | I can distinguish AI applications that already exist from AI applications that are still in the future. |
Technical understanding | I can explain how AI applications make decisions. |
Technical understanding | I can explain how machine learning works at a general level. |
Technical understanding | I can describe how machine learning models are trained, validated, and tested. |
Technical understanding | I can explain the difference between 'supervised learning' and 'unsupervised learning' (in the context of machine learning). |
Technical understanding | I can explain how 'reinforcement learning' works on a basic level (in the context of machine learning). |
Technical understanding | I can explain how deep learning relates to machine learning. |
Technical understanding | I can explain what the term 'artificial neural network' means. |
Practical application | I can critically evaluate the implications of artificial intelligence applications in at least one subject area. |
Critical appraisal | I can explain why data plays an important role in the development and application of artificial intelligence. |
Critical appraisal | I can describe why humans play an important role in the development of artificial intelligence systems. |
Technical understanding | I can describe how some artificial intelligence systems can act in their environment and react to their environment. |
Technical understanding | I can explain how sensors are used by computers to collect data that can be used for AI purposes. |
Practical application | I can name applications in which AI-assisted natural language processing/understanding is used. |
Critical appraisal | I can identify ethical issues surrounding artificial intelligence. |
Technical understanding[11] | I can explain what the term 'black box' means in relation to artificial intelligence systems. |
Technical understanding[12] | I can describe how biases arise in AI systems. |
Critical appraisal | I can critically reflect on the potential impact of artificial intelligence on individuals and society. |
Practical application | I can explain why AI has recently become increasingly important. |
Technical understanding | I can explain how rule-based systems differ from machine learning systems. |
Practical application | I can assess if a problem in my field can and should be solved with artificial intelligence methods. |
Critical appraisal | I can describe what artificial intelligence is. |
Technical understanding | I can describe the concept of explainable AI. |
Critical appraisal | I can explain why data security must be considered when developing and using artificial intelligence applications. |
Critical appraisal | I can explain why data privacy must be considered when developing and using artificial intelligence applications. |
Technical understanding | I can describe the concept of big data. |
Practical application | I can give examples from my daily life (personal or professional) where I might be in contact with artificial intelligence. |
Practical application[13] | I can explain what an algorithm is. |
Critical appraisal | I can describe potential legal problems that may arise when using artificial intelligence. |
[1] This definition and rubric for computational thinking This link will take you to an external website in a new tab. was developed by University of Delaware faculty and staff during a 2016-2018 project funded by the National Science Foundation.
[2] This paper was not identified in the original Web of Science search but is referenced in Southworth et al. (2023). Google Scholar shows that it has 244 citations, comparable to the most frequently cited articles in the Web of Science search results.
[3] Much of this work at the University of Florida predates the introduction of ChatGPT in late 2022.
[4] This paper was also not identified in the original Web of Science search as it was too recent and is "grey literature" (i.e., not published in a peer-reviewed journal).
[5] "Design considerations" were also developed by Long and Magerko but are omitted here.
[6] Had very low communality so dropped from the final model
[7] Loaded on more than one factor so dropped from final model
[8] Loaded on more than one factor so dropped from final model
[9] Loaded on more than one factor so dropped from final model
[10] Loaded on more than one factor so dropped from final model
[11] Had very low communality so dropped from the final model
[12] Loaded on more than one factor so dropped from final model
[13] Loaded on more than one factor and had very low communality so dropped from final model
Guidry, K. R. (2024, October). AI literacy literature summary. University of Delaware Winter Institute on Learning 2025, January 14–16, 2025. Retrieved from https://docs.google.com/document/d/1sjhnmd-CH-k2hOzGGMU_l-cRaSMxTdXLRXzVvU1Sc50/edit?usp=sharing This link will take you to an external website in a new tab.
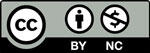